Draft Full Paper Due:
June 1, 2019(Extended)
Notification of Draft Paper Acceptance:
July 1, 2019(Extended)
Author Registration Deadline:
July 15, 2019(Extended)
Final Manuscript Due:
July 15, 2019(Extended)
Regular Attendee Early Bird Registration Deadline:
July 15, 2019(Extended)
High Dimensional Streaming Data Modeling, Monitoring, Diagnosis, and Prognostics
Dr. Jianjun Shi, Member of National of Academy of Engineering (NAE) of USA, Editor-in-Chief of the IISE Transactions, Fellow of IISE, ASME, and INFORMS, The Carolyn J. Stewart Chair and Professor, Georgia Institute of Technology, USA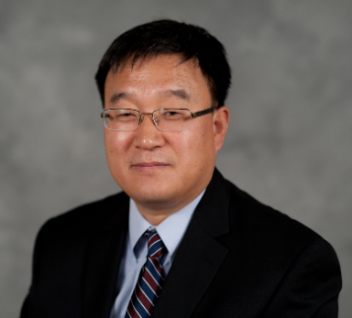
Abstract:
Analysis and modeling of the large-scale high-dimensional streaming data is a very important and challenging problem. This talk focuses on modeling the high-dimensional data obtained from sensors for assessment of system performance, early detection of system anomalies, intelligent sampling and sensing for data collection and decision making to achieve optimal system performance. The developed methodology is efficient and scalable and can be applied for data with complex heterogeneous data structure to extract information or useful features. The talk will discuss six interrelated topics: (i) detect anomalies from high-dimensional image data by using smooth sparse decomposition (SSD), which exploits regularized high-dimensional regression to decompose an image and separate anomalous regions by solving a large-scale optimization problem; (ii) introduce spatial-temporal smooth sparse decomposition (ST-SSD) method for dimension reduction, denoising, and anomaly detection of high dimensional streaming video data; (iii) adaptive sampling for high-dimensional functional data to speed up the inspection and anomaly detection through an intelligent sequential sampling scheme integrated with fast estimation and detection. (iv) dynamic multivariate functional data modeling via sparse subspace learning to achieve automatic data segmentation and grouping for high-dimensional functional data with complex cross-correlation structure. (v) Engineering-driven data analytics for monitoring of multiple functional data, where in-situ Roman sensing data are used to monitoring nanomanufactuirng processes. (vi) A data-level fusion model for developing composite health indices for degradation modeling and prognostic analysis. All those topics presented involve new methodologies development motivated with real engineering problems and validated with real industrial data sets.
Speaker’s Bio:
Dr. Jianjun Shi is the Carolyn J. Stewart Chair and Professor in School of Industrial and Systems Engineering, with a joint appointment in School of Mechanical Engineering, both at Georgia Institute of Technology. He received his B.S. and M.S. in Electrical Engineering from Beijing Institute of Technology in 1984 and 1987, and his Ph.D. in Mechanical Engineering from the University of Michigan in 1992. Dr. Shi’s research is in the area of data enabled manufacturing. His methodologies integrate system informatics, advanced statistics, and control theory, and fuse engineering systems models with data science methods for design and operational improvements of manufacturing and service systems. The technologies developed by Dr. Shi’s research group have been implemented in a wide variety of production systems and produced significant economic impacts. Dr. Shi is the founding chair of the Quality, Statistics and Reliability (QSR) Subdivision at INFORMS, and currently serving as the Editor-in-Chief of the IISE Transactions, the flagship journal of the Institute of Industrial and Systems Engineers (IISE). He is a Fellow of IISE, ASME, and INFORMS, an Academician of the International Academy for Quality, and a member of National of Academy of Engineering (NAE). Dr. Shi received numerous awards for his research and teaching, including the highest recognition in research (IISE David F. Baker Distinguished Research Award) and the highest recognition in education (the IIE Albert G. Holzman Distinguished Educator Award), both from IISE.
Advanced Model Predictive Control (MPC) for Autonomous Underwater Vehicle Systems
Dr. Yang Shi, Fellow of EIC, IEEE, ASME, CSMEProfessor, University of Victoria, Canada
Abstract:
Model predictive control (MPC) is a promising paradigm for high-performance and cost-effective control of complex dynamic systems. This talk will briefly introduce the Ocean Network Center at the University of Victoria. Then an integrated path planning and model predictive tracking control will be presented. Further, the practical problem of dealing with multiple objectives in the control of AUVs will be discussed. Finally, some existing challenges and future research will be analyzed.
Speaker’s Bio:
Yang SHI received the Ph.D. degree in electrical and computer engineering from the University of Alberta, Edmonton, AB, Canada, in 2005. From 2005 to 2009, he was an Assistant Professor and Associate Professor in the Department of Mechanical Engineering, University of Saskatchewan, Saskatoon, Saskatchewan, Canada. In 2009, he joined the University of Victoria, and now he is a Professor in the Department of Mechanical Engineering, University of Victoria, Victoria, British Columbia, Canada. His current research interests include networked and distributed systems, model predictive control (MPC), cyber-physical systems (CPS), robotics and mechatronics, navigation and control of autonomous systems (AUV and UAV), and energy system applications. Dr. Shi received the University of Saskatchewan Student Union Teaching Excellence Award in 2007. At the University of Victoria, he received the Faculty of Engineering Teaching Excellence in 2012, and the Craigdarroch Silver Medal for Excellence in Research in 2015. He received the JSPS Invitation Fellowship (short-term), and was a Visiting Professor at the University of Tokyo during Nov-Dec 2013. His co-authored paper was awarded the 2017 IEEE Transactions on Fuzzy Systems Outstanding Paper Award. He received the Humboldt Research Fellowship for Experienced Researchers in 2018. He is a member of the IEEE IES Administrative Committee during 2017-2019, and the founding Vice Chair of IEEE IES Technical Committee on Industrial Cyber-Physical Systems. Currently, he is Co-Editor-in-Chief for IEEE Transactions on Industrial Electronics; he also serves as Associate Editor for Automatica, IEEE Trans. Control Systems Technology, IEEE/ASME Trans. Mechatrnonics, IEEE Trans. Cybernetics. He is a Fellow of IEEE, ASME and CSME, and a registered Professional Engineer in British Columbia, Canada. He is a Fellow of Engineering Institute of Canada (EIC).
Recent Development and Challenges in Real-Time Machinery Health Condition Monitoring
Dr. Wilson Wang, Fellow of International Society of Engineering Asset ManagementProfessor, Lakehead University, Canada
Abstract:
Reliable online machinery health condition monitoring has become a critical issue in both academic research and industrial applications. Diagnostic information can be used to recognize machinery defect at its earliest stage, so as to prevent machinery performance degradation and malfunction, and improve its operation accuracy and safety. Prognostic information can be applied to estimate the remaining useful life of the faulty component/unit, to properly schedule predictive maintenance operations without unnecessary downtimes so as to reduce costs. In this speech, the related new development and remaining challenges will be discussed in the main areas of real-time machinery health condition monitoring, such as smart sensor networks for data acquisition, signal processing for robust representative feature extraction, and AI-based automatic diagnostics and prognostics. Some issues in implementing a smart monitor for real-time industrial applications in common machinery systems such as pumps, induction motors and gearboxes will also be discussed.
Speaker’s Bio:
Dr. Wilson Wang received his BSc in Electromechanical Engineering (SIT in China), MSc in Mechanical Engineering (Northeastern University in China), MSc in Industrial Engineering (University of Toronto in Canada), and PhD in Mechatronics Engineering (University of Waterloo in Canada) in 2002. From 2002 to 2004, he was a Senior Scientist/Project Manager at Mechworks Systems Inc. He joined Lakehead University (Thunder Bay, Canada) in 2004, where he is currently a Professor in the Department of Mechanical Engineering. His research interests include signal processing, artificial intelligence, machine learning, diagnostics, prognostics, system control, smart sensors, and mechatronics. Dr. Wang is a fellow of International Society of Engineering Asset Management, a senior member of IEEE, and LU Research Chair in Intelligent Systems. He is a receipt of the Distinguished Researcher Award and an Innovation Award. He has supervised 70 highly qualified personnel (PDF, PhD, MSc and MEng students), and published more than 150 peer reviewed articles. He has also successfully developed a series of smart systems for online diagnostics and prognostics of mechanical and electrical machines.
Uncertainty-Based Missile Overall Design Optimization
Dr. Hairui Zhang, Chief Engineer of Series Missile Projects and the Academic Leader of the Aircraft Technology of the Group Company, China Academy of Launch Vehicle Technology, China
Abstract:
With the change of the modern war pattern and innovation of technology, the missile weapon system develops towards the direction of high performance, high reliability and strong survivability. The demands for the optimal overall performance of the missile is ever-increasing. This talk focuses on the application of the uncertainty-based design optimization methodology to the missile overall design in order to solve the difficulties in the new missile development. The talk will discuss four interrelated topics: (i) identify the significant uncertainty parameters greatly impacting on the system response by the max-min distance sequential sampling method, which is combined with the characteristics of missile overall parameter uncertainties on the basis of the Latin hypersonic sample pool; (ii) introduce the improved active learning Kriging reliability analysis method and threshold reliability analysis method to deal with the nonlinear reliability problem and inverse reliability problem respectively, which can significantly enhance the efficiency of the uncertainty analysis in the missile overall design; (iii) present a new uncertainty-based design optimization method based on the margin quantification decoupling strategy, which can improve the efficiency of the missile overall design optimization; (iv) introduce the study of a boost-glide missile overall design, in which the disciplinary models and the corresponding uncertainty models are constructed. The proposed uncertainty-based design optimization method is also employed in this study to achieve the optimal missile overall design. Above topics involving new approaches are demonstrated by the engineering practice.
Speaker’s Bio:
Zhang Hairui received his B.Eng. in aircraft design from Beijing University of Technology in 1997, his M.Eng. in aircraft design from China Academy of Launch Vehicle Technology in 1999, and his Ph.D. from the National Defense University of Science and Technology in 2015. He joined the China Academy of Launch Vehicle Technology in 1999 to engage in aircraft design. He was the Principal Investigator of the development of several missiles. He is currently the Chief Engineer of series missile projects, the Academic Leader of the missile technology of the group company and enjoys the special defense allowance of the state. He has published more than 50 academic papers and 2 monographs.
A Sampled-Data Approach to Analysing Complex Networks
Dr. Zidong Wang, Fellow of IEEEProfessor, Brunel University London, UK
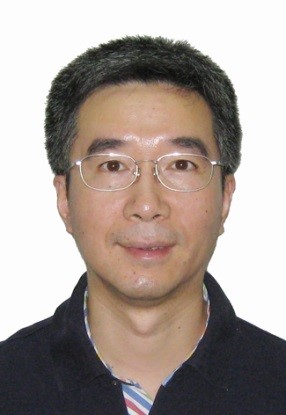
Abstract:
In this talk, we will address the sampled-data synchronization control problem for a class of general complex networks, and then deal with the sampled-data filtering problems for two special classes of complex networks – wireless sensor networks and genetic regulatory networks. The sampling period considered here is time-varying that is allowed to switch between two different values in a random way. The main purpose is to deliver the message that sampled-data issue is vitally important for the applications of complex network theory and the sampled-data filtering/control problems are interesting yet challenging. Both the theoretical research and engineering applications will be discussed, and a series of recently published results will be reported.
Speaker’s Bio:
Professor Zidong Wang is an IEEE Fellow and Professor of Computing at Brunel University London with research interests in intelligent data analysis, statistical signal processing as well as dynamic systems and control. He has been named as the Hottest Scientific Researcher in 2012 in the area of Big Data Analysis. He has been listed as a Highly Cited Researcher in both computer science and engineering science for five consecutive years 2014-2018. He was awarded the AvH Research Fellowship in 1996 from the Alexander von Humboldt Foundation of Germany, the JSPS Research Fellowship in 1998 from the Japan Society for the Promotion of Science and the William Mong Distinguished Research Fellowship in 2002 from the University of Hong Kong. Since 1997, He has published around 220+ papers in IEEE Transactions and 60+ papers in Automatica with an h-index of 111 according to Google Scholar. He is currently serving as the Editor-in-Chief for Neurocomputing, the Deputy Editor-in-Chief for International Journal of Systems Science and an Associate Editor for 10 prestigious journals including 5 IEEE Transactions. His research has been funded by the EU, the Royal Society and the EPSRC of the UK.